The Constructive News Algorithm is an innovative machine learning tool that uses language analysis to identify constructive elements and biases in news coverage. Designed to complement the editorial process, the Constructive News Algorithm helps newsrooms evaluate the “constructiveness” of their coverage.
Get involved
Contact Peter Damgaard Kristensen, COO, Constructive Institute
About the project
How it works
In the newsroom
FAQ
Get weekly editorial reports that show how constructive your reporting has been. We offer a new platform to measure journalistic quality, and the content that matters to your audience.
About the project
A growing number of newsrooms around the world are adopting a constructive approach to journalism. To facilitate this shift, we’ve created an innovative tool to make it easier to identify constructive stories. The Constructive News Algorithm, developed with support from the European Broadcasting Union (EBU) and the Google News Initiative, classifies whether a news article has a constructive narrative or not based on whether its elements align with our standards for constructive journalism: facilitating solutions, nuance and democratic conversation.
The algorithm is fast and consistent, with an 88% accuracy rate compared with our team’s specially trained news raters. It filters articles based on their content — not on clicks, likes or other viral metrics — ensuring it remains a measurable, objective marker for news quality. Our goal is to promote good journalism that helps audiences stay engaged with their communities and the world around them, rather than push them away from the news — and each other.
The Constructive News Algorithm is already being used by news organizations across Europe. And it’s adaptable: News editors can use the tool to streamline their story selection and audit their coverage, giving them a jumping-off point to invest more in constructive angles. Search engines can use it to provide better results, while social media companies can curate the news on their platforms and news consumers can filter for higher quality information using in-browser applications.
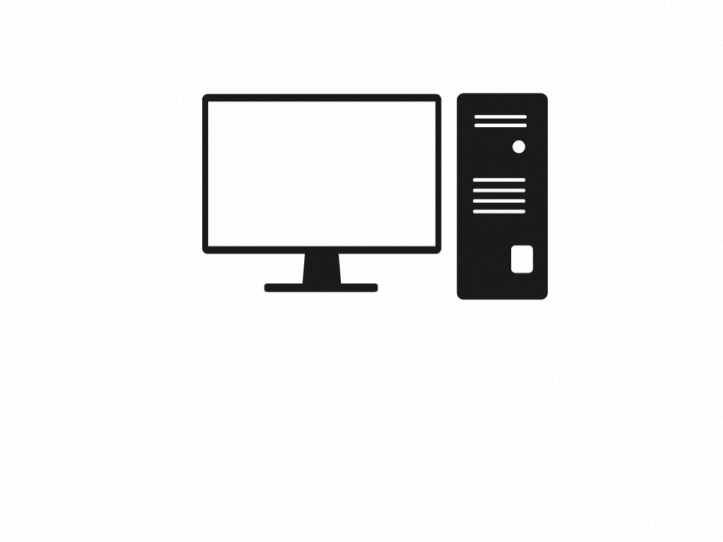
How the algorithm works
In the newsroom: European Broadcasting Union
The European Broadcasting Union — a coalition of public service media organizations in 56 countries — uses the Constructive News Algorithm for its “A European Perspective“ project, which allows member outlets to share news stories from around Europe. Local editors curate stories from the stream of 2,000+ articles every day using subject tags — and now, they can filter for constructive stories identified by the algorithm.
The Constructive News Algorithm makes it easy for editors to quickly identify high quality, constructive stories — and in turn, fulfill the EBU’s public service mission to help audiences contextualize local issues and learn from the experiences of other countries. The first-of-its kind partnership underscores how newsrooms can use artificial intelligence tools to spread high-quality, trusted journalism and foster collaborations across borders.
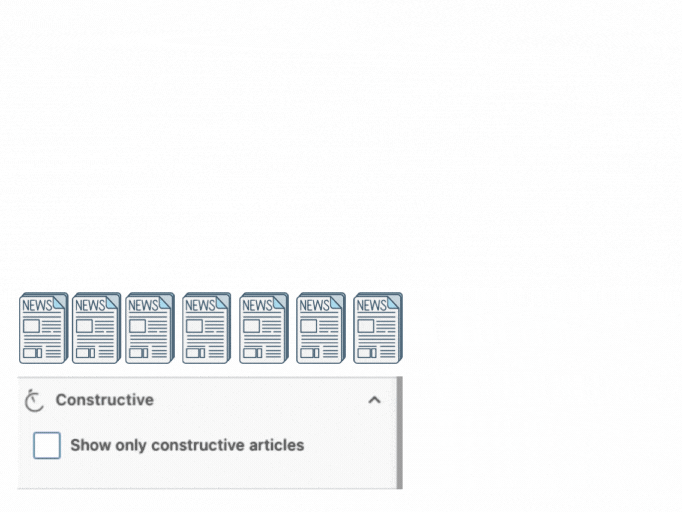
The Constructive News Algorithm is an exciting addition to our digital news hub. The algorithm has been developed by the Constructive Institute with important input from our members. It provides an additional important insight into our journalism that inspires new ideas and seeing challenges from different perspectives. This is particularly important for public service media and its contribution to society.
Justyna Kurczabinska, who heads the EBU’s News Strategy and Transformation of Eurovision News
Frequently Asked Questions
The Constructive Institute has been developing the News Algorithm since 2019, with support from the Google News Initiative and the European Broadcasting Union’s “A European Perspective” project, co-financed by the European Union’s Preparatory Action – “European Media Platforms.”
We used articles from large Danish news organizations and members of the EBU’s “A European Perspective” project. We included both web and print news articles, and omitted obituaries, interviews, sports stories, etc., in order to optimize the dataset for our specific use case. This also means that the model does not handle these types of articles. In total, 28 news media contributed articles for the dataset. All articles were translated to English using DeepL.
With the field of language models constantly evolving, we are quickly adapting our algorithm for many different use cases. We believe that the advancements in language technology will allow us to create a set of tools to help news editors to monitor their output — not only by the clicks they generate, but also the editorial values that shape responsible journalism.
The Constructive News Algorithm is designed to complement and streamline journalists’ editorial processes by enabling them to audit their own coverage for constructive elements.
A group of primarily Danish Fellows and employees from the Constructive Institute did the initial coding of more than 3,000 news articles, which was used to train the Constructive News Algorithm. We’re always looking to expand our dataset of news stories as well as our coding team to ensure our algorithm takes into account a wide variety of perspectives.
We are currently testing the Constructive News Algorithm with multiple newsrooms. If you’d like to get involved or have questions, please reach out to COO, Constructive Institute, Peter Damgaard Kristensen.

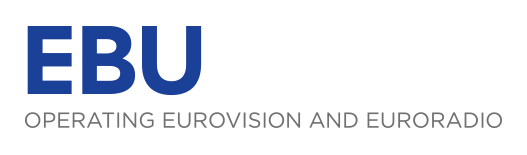
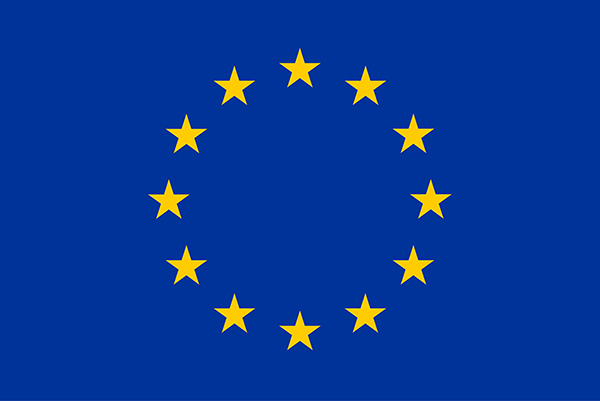